In the insurance industry, rule engines are very common for automating decision-making at various stages.
Introduction
- In the insurance industry, rule engines are very common for automating decision-making at various stages. Rule Engines are widely used in various parts of insurance, such as Buying Journeys, Claims, Underwriting, Personalization, Rewards, and Endorsements.
Benefits in the Insurance Industry
1. Efficiency and Speed
- Automates routine decisions, speeding up processes such as underwriting and claims processing.
2. Consistency
- Ensures decisions are made consistently across similar cases, improving fairness and customer satisfaction.
3. Compliance
- Helps in adhering to regulations by incorporating legal and policy rules directly into the decision-making process.
4. Scalability
- Can handle a large volume of cases without additional cost, improving the scalability of insurance operations.
5. Adaptability
- Allows quick updates to rules in response to changes in laws, market conditions, or company policies.
Problems with Rule Engines
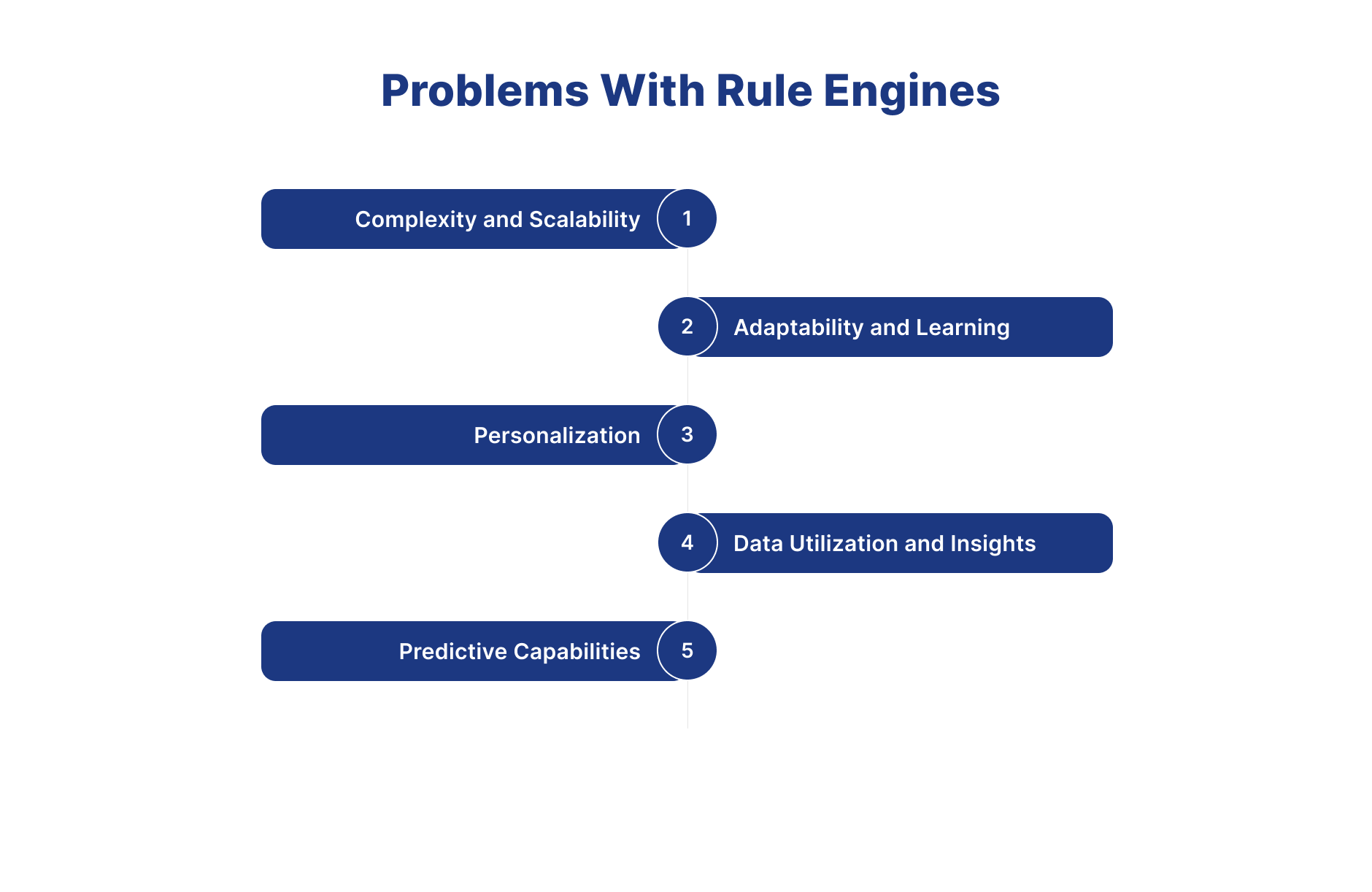
- Rule Engines works by business rules configured on the system. Each rule needs to be reviewed by the maker and checker. If
1. Complexity and Scalability
- As the number of rules increases, the system can become very complex, making it difficult to manage, understand, and update the rules. The interdependencies between rules can lead to unexpected outcomes or conflicts that are hard to diagnose and resolve.
2. Adaptability and Learning
- Rule engines operate on a fixed set of predefined rules. They cannot learn from new data or adapt to changing patterns in customer behavior, market conditions, or fraud tactics without manual intervention to update the rules.
3. Personalization
- While rule engines can make decisions based on predefined criteria, they may not be as effective in personalizing these decisions or offers to individual customers’ preferences or behaviors because they do not learn from individual interactions.
4. Data Utilization and Insights
- They are typically limited to using structured data and specific inputs defined by the rules. This can limit the depth of analysis and the ability to uncover insights from unstructured data sources, such as text or images.
5. Predictive Capabilities
- Rule engines are inherently deterministic and are not designed to predict future outcomes beyond the scenarios covered by the existing rules.
How can AI help?
- While rule engines play a significant role in automating decision-making processes in the insurance industry, their capabilities can be limited compared to the more dynamic and adaptive nature of Artificial Intelligence (AI). Here are some of the solutions AI can help:
1. Complexity and Scalability
-
AI models, especially machine learning-based ones,can automatically adjust to new data and learn from outcomes, reducing the complexity of manually managing a large set of rules.
-
AI models can also identify inter-connected effects of the inputs.
2. Adaptability and Learning
- AI and machine learning models thrive on new data, continuously improving and adapting their predictions or decisions based on the latest information, trends, and outcomes.
3. Personalization
- Massive volumes of data may be analysed by AI at an individual level, allowing for highly personalized recommendations, pricing, and products tailored to each customer's unique needs and risk profiles.
- For example, in claims, instead of identifying the standard rules, AI can identify many patterns to justify whether a claim is legitimate.
- Underwriting can also identify hidden risks with AI, compared to a rule engine, where new rules need to be added regularly.
4. Data Utilization and Insights
-
AI can process and analyze structured and unstructured data, uncovering deeper insights and patterns that may not be evident through rule-based analysis. This capability enhances risk assessment, fraud detection, and customer service.
-
Complex multi-group conditions and interconnected rules can be difficult to configure in rule engines. However, in the case of AI, models can be trained with multiple parameters and can adapt to them effectively.
5. Predictive Capabilities
- AI models, especially those using machine learning, have strong predictive capabilities, allowing them to forecast future trends, anomalies, and potential risks or opportunities.
Note: AI can be much more helpful once the data size is huge and there are a huge number of patterns. AI can also be leveraged to find hidden rules or patterns in data.
When to use AI over Rule Engines?
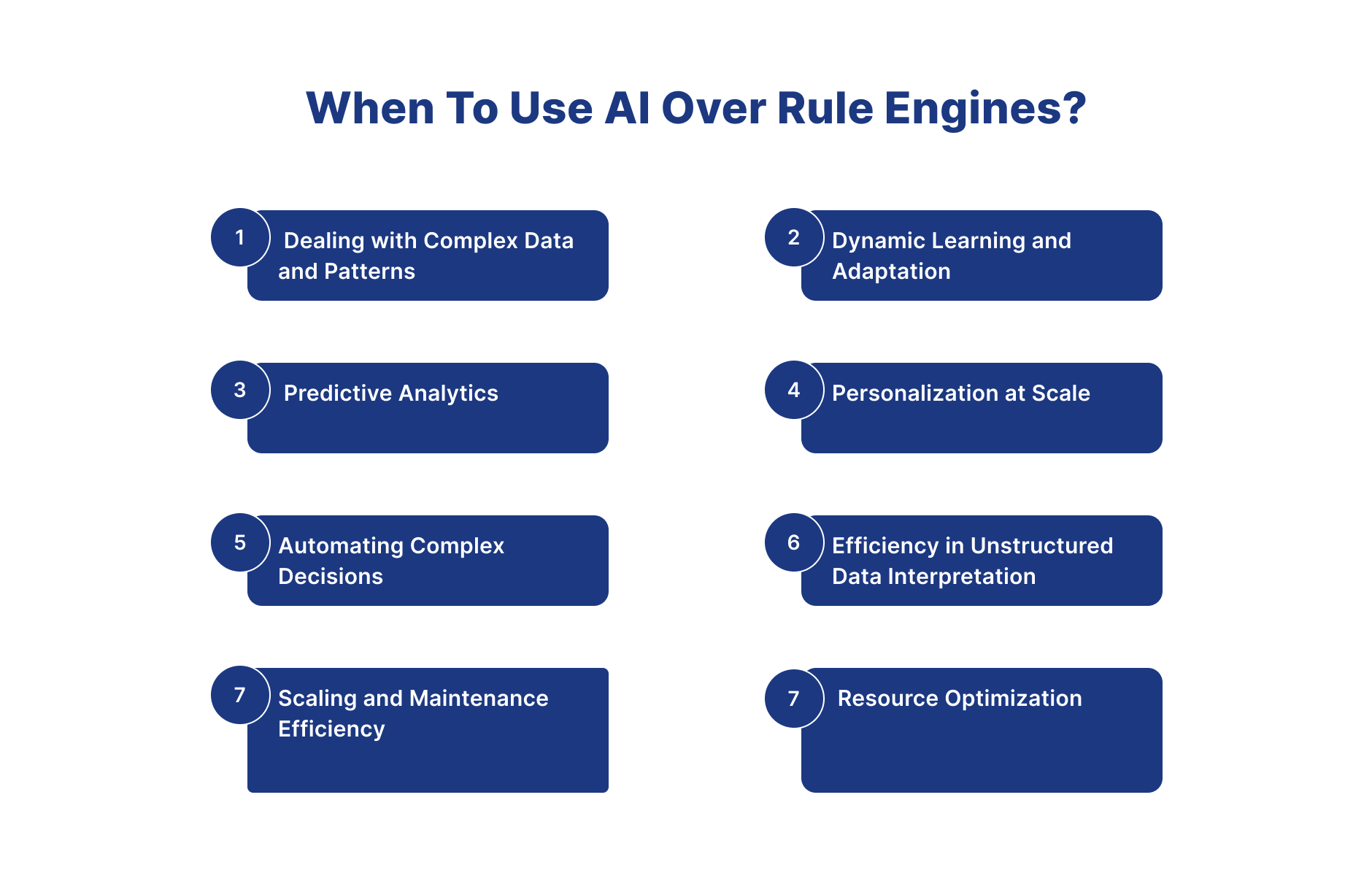
1. Dealing with Complex Data and Patterns
- AI can provide significant advantages when the data involved is vast, unstructured, or too complex for manual rule setting. AI, particularly machine learning, can identify patterns and correlations within large datasets that would be impractical to decipher manually or with a rule-based system.
2. Dynamic Learning and Adaptation
- AI systems can continuously learn and adapt when the environment is constantly changing, such as evolving market trends, consumer behaviors, or fraud tactics. Unlike rule engines that require manual updates to rules, AI models can automatically adjust based on new data, ensuring decisions remain relevant and accurate.
3. Predictive Analytics
- AI's predictive capabilities are far superior for forecasting future events or behaviors, such as predicting which policies might lead to claims or identifying high-risk customers. Rule engines operate on predefined logic and cannot forecast future results using patterns in previous data like AI models can.
4. Personalization at Scale
- AI excels at delivering personalized experiences, recommendations, or products by analyzing individual customer data. This level of personalization is challenging to achieve with rule engines, especially at a large scale, due to the complexity of creating and managing the necessary rules for each customer scenario.
5. Automating Complex Decisions
- When decisions involve multiple factors or dependencies or require balancing trade-offs in non-straightforward ways, AI can evaluate all aspects in a nuanced manner. Rule engines might be too rigid or simplistic for these complex decision-making processes.
6. Efficiency in Unstructured Data Interpretation
- Through natural language processing (NLP) and computer vision, AI can interpret unstructured data such as text, images, and audio. This capability is particularly useful for processing claims, analyzing customer feedback, or assessing risk from photographs or documents, which would be beyond the capabilities of a rule engine.
7. Scaling and Maintenance Efficiency
- As the number of rules grows in a rule engine, so does the complexity and difficulty in maintaining accuracy and performance. AI models, especially machine learning-based ones, can scale more efficiently as they learn from additional data without a proportional increase in complexity or maintenance effort.
8. Resource Optimization
- In scenarios where optimizing resources is critical—such as dynamically pricing policies based on risk or customer value AI can analyze numerous factors to optimize decisions in real time, something that would be cumbersome and less effective if attempted through static rules.
How do transition from Rule Engine to AI?
1. Assessment and Planning
-
Evaluate Current Capabilities: Assess the existing rule engine infrastructure, including the complexity of rules, performance, and areas where it falls short in efficiency or effectiveness.
-
Identify Objectives: Define what you aim to achieve with AI, such as improved decision accuracy, personalized customer experiences, or enhanced predictive analytics.
-
Skill Gap Analysis: Determine the skills available within your organization and identify gaps. Using AI to train current employees or hire new talent and machine learning expertise may be necessary.
2. Data Infrastructure and Management
-
Data Accessibility: Ensure that relevant data is accessible and organized. In order to train, AI models require a lot information high-quality data.
-
Data Quality and Integration: Improve data collection and processing practices to ensure data quality. Integrate disparate data sources to provide a holistic view of the AI models.
3. Pilot Projects
-
Select Pilot Areas: Choose specific processes or products where AI can significantly impact. Pilot projects should be manageable in scope and offer clear metrics for success.
-
Prototype Development: Develop AI prototypes for these selected areas. This step often involves choosing the right algorithms, training models with historical data, and integrating AI predictions or decisions with existing workflows.
4. Integration and Testing
-
Integration: Carefully integrate AI models with the existing IT infrastructure, ensuring they can access the necessary data and interact with other systems.
-
Testing and Validation: Rigorously test the AI models to validate their decisions against historical outcomes and ensure they meet regulatory and ethical standards. Testing should also include performance, scalability, and reliability assessments.
5. Training and Change Management
-
Staff Training: Train staff on how AI models work, interpret their outputs, and manage or override decisions when necessary.
-
Change Management: Use techniques for managing change to deal with opposition. and ensure a smooth transition. Communicate the benefits of AI and involve key stakeholders in the transition process.
6. Scaling and Continuous Improvement
-
Expand Use Cases: Based on the successes of the pilot projects, gradually expand the application of AI to additional processes and products.
-
Continuous Learning and Improvement: AI models should continuously learn from new data and outcomes. Regularly retrain models to adapt to changes in the market or business environment.
-
Feedback Loops: Establish mechanisms for continuous feedback on AI performance, incorporating insights from users and stakeholders to refine AI models and their integration with business processes.
7. Regulatory Compliance and Ethical Considerations
-
Compliance: Ensure AI applications comply with all relevant regulations and industry standards, particularly concerning data privacy, consumer protection, and nondiscrimination.
-
Ethical AI Use: Develop guidelines for ethical AI use, addressing potential biases in AI models and ensuring transparency and fairness in AI-driven decisions.
AI models and architectures can be used as alternatives to the rule engine.
1. Machine Learning (ML) Models
-
Overview: ML models learn from historical data to forecast or decide without the need for explicit programming with rules. Some well-known algorithms are Decision Trees and Random Forests, Support Vector Machines (SVM), etc. Multi-model approaches can also be used.
-
Applications: Underwriting by predicting risk levels, processing claims by automating approval decisions based on historical claims data, and detecting fraud through pattern recognition.
2. Deep Learning (DL) Models
-
Overview: A subset of ML, deep learning use deep networks, or multilayered neural networks, to learncomplex patterns in large amounts of data. Convolutional Neural Networks (CNNs), Recurrent Neural Networks (RNNs), Long Short-Term Memory (LSTM) Networks, Transformer Models, Autoencoders, etc. can be used.
-
Applications: Natural language processing for understanding customer inquiries automatically and personalizing customer interactions based on deep customer behavior analysis, underwriting risk identification, and claims anomaly detection.
3. LLM
-
Overview: Unlike rule engines that rely on predefined logic and strict if-then rules, LLMs can understand, interpret, generate, and process natural language at a high level of sophistication. This capability enables them to handle various tasks that require understanding context, making inferences, and learning from vast amounts of unstructured data.
-
Applications: Document Processing and Analysis, Risk Assessment and Policy Underwriting, Claims Processing etc.
Conclusion
- In conclusion, the traditional use of rule engines in the insurance industry has facilitated automation, bringing efficiency, consistency, scalability, and regulatory compliance to various operations like buying journeys, claims processing, underwriting, and customer personalization. However, as the complexity of data and consumer demands evolve, the limitations of rule engines in scalability, adaptability, personalization, and predictive analytics become apparent. This is where Artificial Intelligence (AI) shines, offering dynamic, learning-driven alternatives that can handle complex patterns, provide personalized experiences at scale, and utilize structured and unstructured data for deeper insights.